Unlock Insights, Drive Decisions
Empowering Your Business with Data Mining
Excellence.
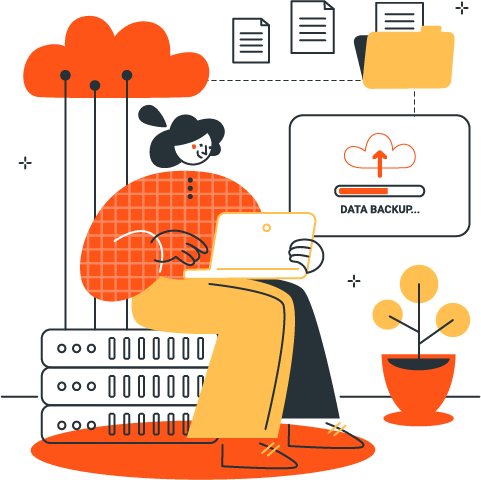
Data Mining Services
In today’s data-driven economy, the capacity to analyze and extract actionable insights from vast amounts of information is vital for strategic decision-making. Data mining services, which focus on discovering patterns and knowledge from extensive datasets, empower organizations to make informed choices, optimize processes, and enhance customer experiences. As businesses navigate increasingly complex data landscapes, the significance of data mining has never been more pronounced.
This article explores the importance, techniques, components, challenges, and best practices associated with data mining services, offering a comprehensive overview for organizations eager to harness the power of their data.
Importance of Data Mining
Data mining serves as an essential tool for extracting valuable insights from large volumes of structured and unstructured data. Organizations across various sectors leverage data mining to identify trends, understand customer behavior, improve operational efficiency, and foster innovation.

Enhanced Decision-Making
Data mining provides businesses with the insights needed for data-driven decision-making. For example, retail companies can analyze customer purchasing patterns to tailor their marketing strategies, resulting in improved sales and increased customer loyalty.
Predictive Analytics
Employing data mining techniques enables predictive analytics, allowing organizations to forecast future trends based on historical data. This capability is particularly beneficial in industries like finance, where firms can assess risk and make informed investment choices.
Improved Customer Insights
Understanding customer preferences and behaviors is crucial for crafting effective marketing campaigns. Data mining facilitates customer segmentation, enabling businesses to create targeted promotions and enhance overall customer satisfaction.
Fraud Detection and Risk Management
In sectors such as banking and insurance, data mining plays a critical role in identifying fraudulent activities and managing risks. By analyzing transaction patterns, organizations can detect anomalies and mitigate potential losses.
Operational Efficiency
Data mining helps organizations identify inefficiencies in their processes and workflows. By streamlining operations, businesses can achieve cost savings and improved productivity, ultimately enhancing their bottom line.
Competitive Advantage
Data mining provides businesses with the insights needed for data-driven decision-making. For example, retail companies can analyze customer purchasing patterns to tailor their marketing strategies, resulting in improved sales and increased customer loyalty.
Key Components of Data Mining Services
Data mining is a multifaceted process involving several key components that contribute to its overall effectiveness.
Data Collection
The foundation of data mining lies in robust data collection. Organizations gather data from various sources, including databases, social media, customer feedback, and transactional records. The quality and relevance of the collected data are critical for successful analyses.
Data Cleaning and Preparation
Data is often messy and inconsistent, necessitating thorough cleaning and preparation before analysis. This step includes removing duplicates, correcting errors, and addressing missing values. Properly prepared data enhances the accuracy of the generated insights.
Data Exploration and Visualization
Exploratory Data Analysis (EDA) is essential for understanding the characteristics of the data. Visualization tools, such as graphs and charts, help analysts identify trends, correlations, and outliers within the dataset. This exploratory phase lays the groundwork for more sophisticated analytical techniques.
Data Mining Techniques
Data mining techniques like Classification, Clustering, Association Rule Learning, and Anomaly Detection serve unique analytical goals—categorizing data, segmenting markets, discovering item relationships, and detecting irregular patterns, respectively—enabling organizations to gain valuable insights.
Model Evaluation and Validation
After developing a data mining model, evaluating its performance is essential. Techniques such as cross-validation, confusion matrices, and ROC curves help determine the model’s accuracy and reliability. A well-validated model ensures that the derived insights are both actionable and trustworthy.
Deployment and Monitoring
Once a model is validated, it can be deployed in a production environment. Continuous monitoring is vital to ensure the model adapts to new data and remains relevant over time. This phase may involve retraining the model as new data becomes available.
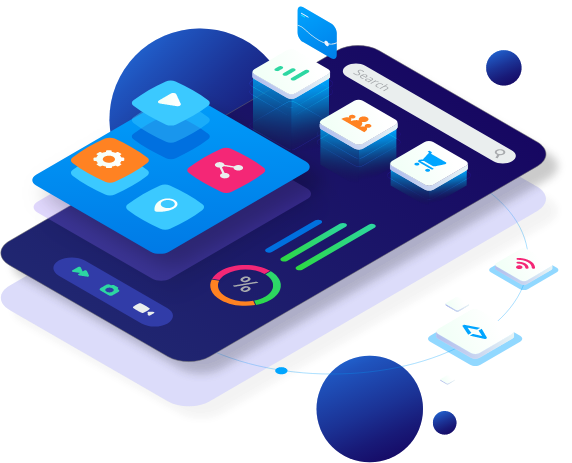
Challenges in Data Mining
Data mining faces several significant challenges that can hinder the extraction of valuable insights from large datasets. One major issue is data quality, as inaccurate, incomplete, or inconsistent data can lead to misleading results, necessitating thorough preprocessing. Additionally, the sheer volume and variety of data generated today complicate effective processing and analysis, requiring robust storage solutions and the ability to handle different data formats.
Privacy and security concerns, particularly regarding sensitive information, add another layer of complexity, especially with regulations like GDPR. Furthermore, ensuring model interpretability, avoiding overfitting, and adapting to changing data patterns are critical for maintaining accuracy. The selection of appropriate algorithms, managing computational complexity, and addressing noise and outliers are also vital for successful data mining, making it a multifaceted and challenging endeavor.
Understanding and Solving Major Challenges in Data Mining Processes
1. Data Quality Issues
The quality of data is paramount in data mining. Poor-quality data, characterized by inaccuracies, inconsistencies, or incompleteness, can lead to misleading results. Organizations must invest in robust data governance practices to ensure data integrity.
2. Privacy and Security Concerns
Data mining often involves handling sensitive information, raising significant privacy and security concerns. Organizations must comply with data protection regulations such as GDPR or CCPA to safeguard customer information and avoid legal repercussions.
3.Complexity of Data
As data sources become increasingly diverse and complex, organizations face challenges in integrating and analyzing these datasets. Advanced tools and technologies, such as artificial intelligence and machine learning, are essential for effectively processing large volumes of data.
4. Skills Gap
The demand for skilled data scientists and analysts continues to outpace supply. Organizations may struggle to find professionals with expertise in data mining techniques and statistical analysis, hindering their ability to leverage data effectively.
5. Interpreting Results
Extracting insights from data is only part of the challenge; interpreting those insights and translating them into actionable strategies is equally important. Organizations must ensure their teams can effectively communicate findings and integrate them into decision-making processes.
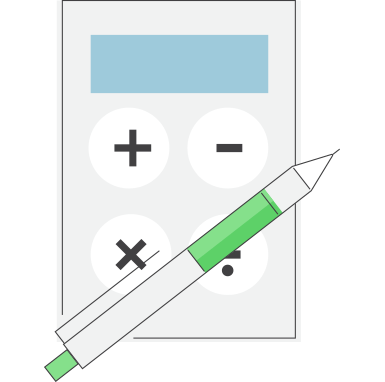
Leverage Advanced Tools and Technologies
Utilizing modern data mining tools and software can streamline the analysis process. Tools like Python, R, and specialized data mining platforms can enhance the efficiency and effectiveness of data mining initiatives.
Best Practices in Data Mining
To maximize the effectiveness of data mining services, organizations should adhere to several best practices. First, it is essential to ensure data quality by implementing robust data cleaning and preprocessing techniques to eliminate inaccuracies and inconsistencies. Second, organizations should define clear objectives and key performance indicators .
Define Clear Objectives
Establishing clear goals for data mining efforts is essential. Organizations should outline the specific questions they aim to answer or the problems they intend to solve through data analysis. This clarity will guide the entire data mining process.
Invest in Quality Data
Ensuring high-quality data is fundamental for effective data mining. Organizations should implement data governance frameworks that prioritize data accuracy, consistency, and reliability. Regular data audits and cleaning processes can help maintain data quality.
Foster a Data-Driven Culture
Encouraging a culture of data-driven decision-making across the organization can significantly enhance the impact of data mining efforts. Providing training and resources for employees at all levels fosters a greater understanding of data's value.
Continuous Learning and Adaptation
The field of data mining is dynamic, with new techniques and technologies continually emerging. Organizations should prioritize continuous learning and adaptation to keep their teams informed about the latest advancements and best practices.
Conclusion
Data mining services are indispensable in today’s rapidly evolving business landscape. By effectively analyzing vast amounts of data, organizations can uncover valuable insights that drive strategic decision-making, enhance operational efficiency, and foster innovation. As businesses continue to navigate the complexities of big data, investing in robust data mining services will be critical for those seeking to harness the full potential of their data assets.
With a clear understanding of the importance, techniques, challenges, and best practices associated with data mining, organizations can position themselves as leaders in their respective industries. By leveraging data mining to its fullest, businesses can transform raw data into actionable insights, ultimately leading to improved outcomes and sustained growth.
Unlock the Power of Data Mining Today!
Are you ready to take your data analysis to the next level? By implementing best practices in data mining, you can uncover valuable insights and drive strategic decision-making in your organization.